Demystifying Explainable AI: Lifting the Lid on AI’s Thinking
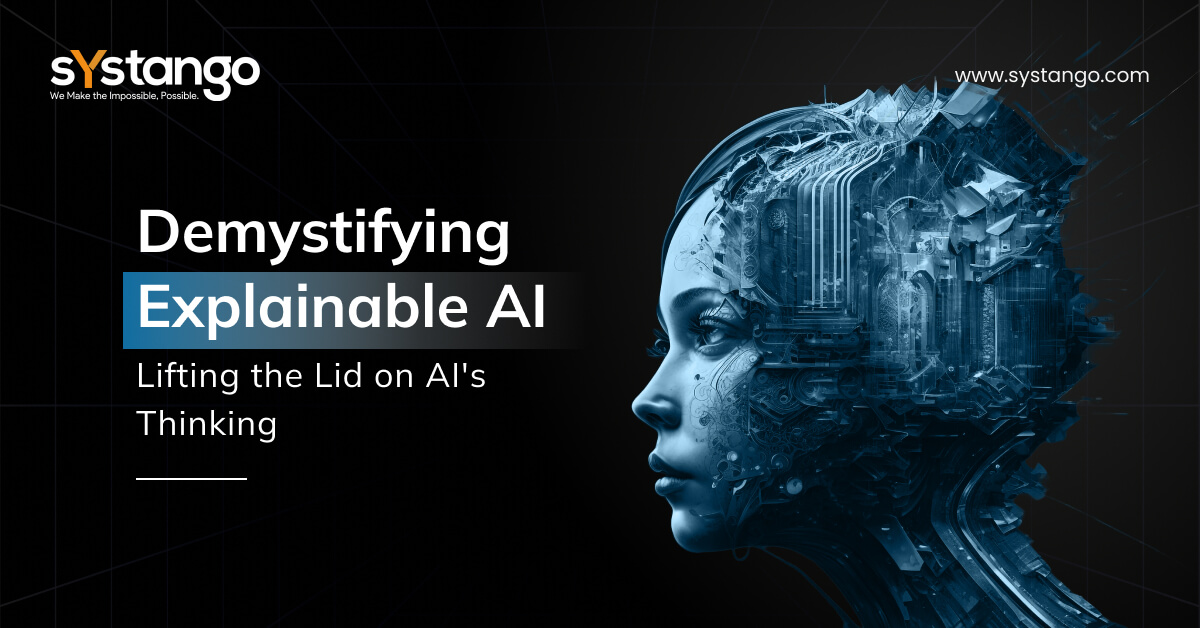
Imagine this –> you walk into a busy hospital, seeking a crucial diagnosis. The latest AI technology analyses your scans, and in a flash, a diagnosis appears on the screen. But what if that diagnosis feels eerily wrong? You ask for an explanation, only to be met with a silent machine – a black box of algorithms crunching numbers in a language you don’t understand. This is the conundrum of modern AI.
And here is when Explainable AI comes in as a rescuer.
We all know that AI today is revolutionising everything from healthcare to finance, and transportation to entertainment. Its algorithms are weaving themselves into the fabric of our lives, yet their inner workings remain covered in mystery. We entrust them with vital decisions, but how do they arrive at those conclusions? Are they truly objective, or could subtle biases be lurking beneath the surface?
Beyond the Buzzwords
AI, once the stuff of science fiction, now permeates every facet of our lives. Yet, as these models grow in complexity, so too does the unease around their inner workings. This is where Explainable AI (XAI) enters the stage. It’s like pulling back the curtain on the wizard, shedding light on the hidden workings of AI models. It’s about unlocking the black box, turning opaque algorithms into transparent windows that reveal how they arrive at their decisions.
The Black Box Unboxed
Black-box models, in the context of AI and machine learning, are models whose internal workings are difficult or impossible to understand directly. They produce accurate predictions or outputs, but we can’t easily see how they arrive at those conclusions.
Here are some key characteristics of black-box models:
- Complex algorithms: Often based on deep neural networks or other intricate mathematical formulations, making them difficult to decipher directly.
- Large datasets: Trained on massive amounts of data, which further obscures the individual contributions of each data point to the model’s predictions.
- Non-linear relationships: Many black-box models capture complex relationships between features and predictions that are not easily expressed in straightforward formulas.
Importance of Explainable AI
Let’s understand it with 2 examples –
Take, for instance, the case of a loan application denied by an AI-powered system. Without XAI, the applicant is left in the dark, grappling with feelings of injustice and frustration. They have no way of knowing whether the rejection was based on valid financial metrics or hidden biases lurking within the algorithm. With XAI, the applicant can see the specific factors that influenced the decision, opening the door to potential appeals or adjustments.
The need for Explainable AI goes far beyond individual anecdotes. Here is one of the most prominent Explainable AI examples. Imagine a self-driving car making a sudden, life-altering decision in split seconds. Understanding its reasoning becomes crucial not just for passenger safety but also for legal and ethical accountability. In healthcare, a misdiagnosis based on an unexplained AI finding could have devastating consequences.
Unleashing the Power of Partnership
Explainable AI helps in bridging the gap between the cold logic of machines and the warm, messy reality of human lives.
Humans excel at creativity, empathy, and critical thinking, while AI possesses unparalleled computational power and the ability to analyse vast amounts of data. When we combine these strengths, we unlock a future where AI serves as a powerful tool for good, its potential unconstrained by the shackles of opacity.
“XAI is about ensuring that the AI revolution doesn’t leave us in the dark but empowers us to understand, collaborate with, and ultimately harness the power of these intelligent machines for the good of all.”
Now that you have some idea about What is Explainable AI before we proceed and understand XAI in detail. We are curious to know from you – what ethical concerns you have about the development and use of Explainable AI. (Let’s spark a conversation in the comments below!)
What is XAI? Unlocking the “Why” Behind the “What”
We’ve peered behind the curtain and witnessed the dazzling feats of XAI.
But how does it perform these wonders?
Let’s understand Explainable AI (XAI) – the Rosetta Stone for AI, translating the arcane language of algorithms into something we can grasp – in detail.
The main concept that distinguishes XAI from AI is the emphasis on explainability. While AI can involve both understanding the model itself (interpretability) and why it makes certain decisions (explainability), XAI specifically focuses on providing explanations for the model’s predictions.
Interpretability vs Explainability in AI
While both “Interpretability” and “Explainability” deal with making AI understandable, they differ in nuance.
- AI Interpretability focuses on the technical workings of a model, like exploring the ingredients and steps in a recipe. Explainability, on the other hand, is about the “why” – the specific factors that influence the outcome, like helping you understand why a dish turned out salty or sweet.
- Imagine, for example, a bank using AI to assess loan applications. Interpretability would break down the model’s algorithms, but XAI would reveal the specific financial metrics that tipped the scales for or against an applicant. This transparency fosters trust, allowing for appeals and adjustments if needed.
Now that you know about Explainable AI in more depth, let’s move on to understand the principles behind the operation of XAI.
Explainable AI Principles: Building Trust and Understanding
Explainable AI (XAI) goes beyond revealing AI decisions; it’s about cultivating trust and collaboration between humans and machines. Key Explainable AI principles include:
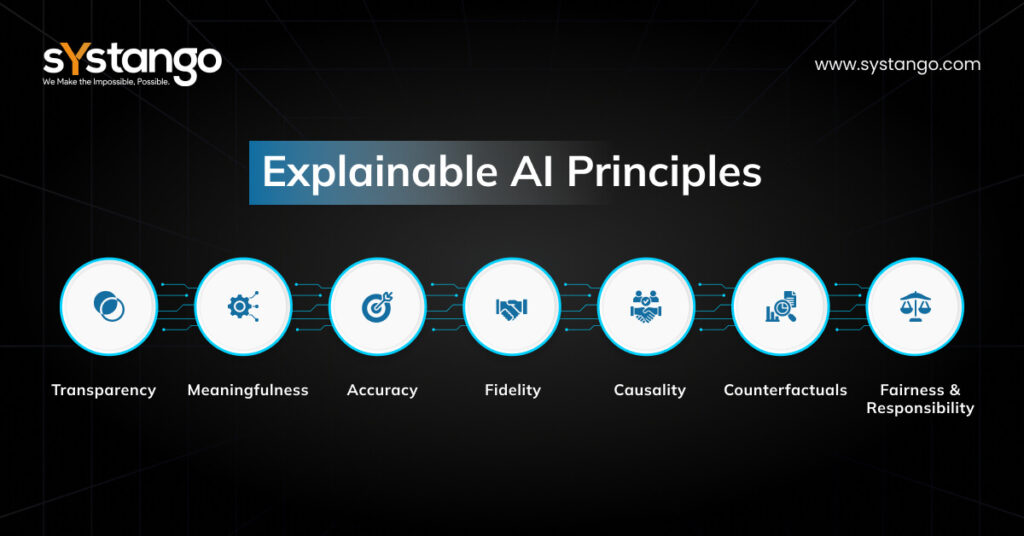
1. Transparency
AI decisions are clarified, revealing not just outcomes but also the influencing factors.
2. Meaningfulness
Explanations are tailored to the audience, avoiding technical jargon for ordinary users.
3. Accuracy
Truthful explanations build trust; misleading information can have unintended consequences.
4. Fidelity
Explanations capture the AI model’s complexity without oversimplification, ensuring a comprehensive understanding.
5. Causality
Uncovering causal relationships between inputs and outputs provides insights into decision-making.
6. Counterfactuals
Exploring “what-if” scenarios helps understand how changes impact outcomes, aiding better decision-making.
7. Fairness and Responsibility
XAI identifies and addresses biases, promoting fair and responsible AI.
These Explainable AI principles guide XAI development, ensuring it builds trust, promotes collaboration, and unleashes AI’s potential responsibly. XAI isn’t a magic bullet, but a tool; applying its principles fosters a future where humans and machines collaborate for a better tomorrow.
Benefits of Explainable AI (XAI)
Ever feel bamboozled by an AI’s decision, left wondering why it chose option A over B? That’s where Explainable AI (XAI) shines, lifting the veil on these enigmatic choices and unleashing a cascade of benefits:
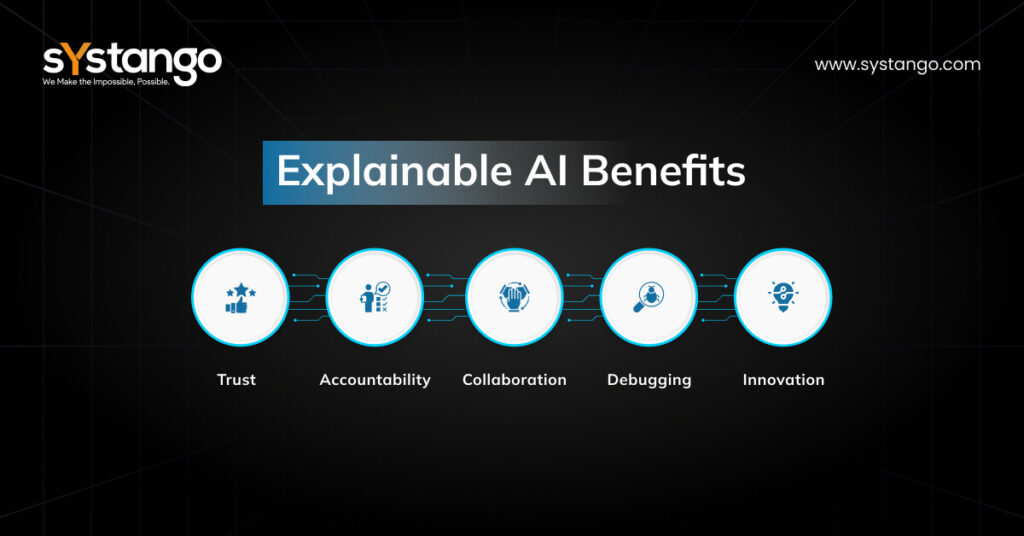
1. Trust
With XAI, AI decisions aren’t black boxes anymore. You understand the “why” behind the “what,” fostering trust and confidence in AI, making it a true partner, not a shrouded oracle.
2. Accountability
Unmasking AI’s logic allows for accountability and responsible development. No more biased algorithms or unfair outcomes hiding in the shadows. XAI shines a light, ensuring decisions are fair, ethical, and transparent.
3. Collaboration
When you understand AI, you can collaborate with it. XAI allows humans and AI to work together, leveraging each other’s strengths. Humans provide domain knowledge and ethical guidance, while AI crunches data and offers insights. This synergy is the true magic of XAI.
4. Debugging
Imagine wrestling with a malfunctioning washing machine – confusing lights, and cryptic codes. XAI Artificial Intelligence is like the expert repair person, pinpointing the problem with ease. By explaining how AI decisions are made, XAI simplifies debugging, leading to faster improvements and smoother performance.
5. Innovation
Transparency inspires innovation. With XAI, developers can experiment, refine, and push the boundaries of AI, knowing the impact of each tweak. This open feedback loop allows AI to evolve, adapt, and ultimately, deliver even greater value.
These are just a few of the countless benefits XAI offers. From building trust to fostering collaboration, XAI is the essential ingredient that unlocks the true potential of AI, transforming it from a cryptic puzzle to a powerful tool for progress.
Unveiling the Secrets: A Guide to XAI’s Diverse Toolkit
Like a skilled detective unravelling a mystery, Explainable AI Software employs a variety of techniques to illuminate AI’s inner workings. Let’s explore two key distinctions within this toolkit: model-centric versus data-centric approaches, and model-specific versus model-agnostic techniques.
Let’s delve into the details of the Explainable AI Models with an example.
Imagine you’re exploring a fascinating city trying to understand everything that makes it beautiful.
Navigating the AI Landscape: Model-Centric vs. Data-Centric
Here are the details –
Model-centric explainability | Data-centric explainability |
It focuses on understanding the city’s architecture—its streets, buildings, and landmarks. It delves into the model’s structure, logic, and decision-making processes. | It explores the city’s inhabitants and their stories. It examines the data that powers the model. |
Key features: These are the influential elements shaping predictions, like a city’s most prominent landmarks. | Data patterns and relationships: Uncovering hidden connections and trends, like identifying popular neighbourhoods and cultural hubs. |
Input interactions: How the model responds to different inputs, like a city’s traffic patterns adapting to rush hour. | Data’s influence on predictions: Understanding how data shapes model outcomes, like how a city’s history influences its present character. |
Sensitivity to data changes: How the model’s behaviour adapts to variations in data, like a city’s resilience to weather events. | Potential biases and inconsistencies: Detecting unfair or misleading patterns in the data, like addressing social inequalities within the city. |
Together, these approaches create a comprehensive understanding of AI’s decision-making processes, ensuring trust and accountability.
Tailored Solutions vs. Universal Tools: Model-Specific vs. Model-Agnostic
Now, let’s consider how XAI techniques interact with different model types.
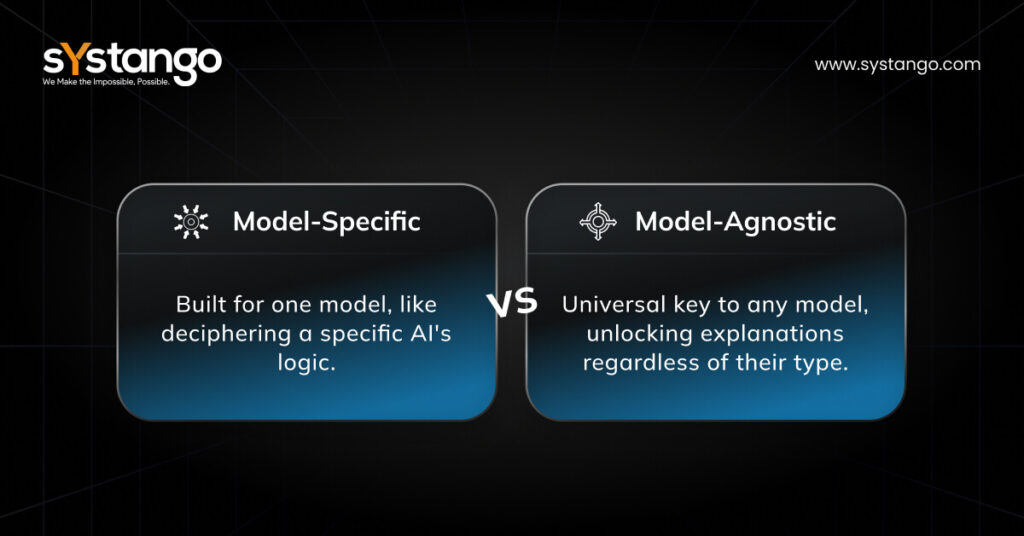
Model-Specific vs. Model-Agnostic
Model-specific: Built for one model, like deciphering a specific AI’s logic.
Model-agnostic: Universal key to any model, unlocking explanations regardless of their type.
Model-specific explainability
These techniques are custom-designed for particular AI model architectures, like a city guide specialising in historical tours. They offer in-depth insights into specific models, but may not apply to others.
Model-agnostic explainability
These techniques are adaptable explorers, able to navigate diverse model types, like a versatile city guide who can cater to any interest. They provide general explanations that can be applied to a wider range of AI models.
By strategically employing these techniques, XAI practitioners can illuminate AI’s reasoning, fostering trust and ensuring responsible AI development. It’s like having a trusted guide who can navigate the complexities of AI, making its decisions transparent and understandable to all.
LIME and SHAP: Soloists in the XAI Orchestra
Within this symphony, two techniques stand out as star performers:
LIME (Local Interpretable Model-agnostic Explanations)
Lime Explainable AI is a versatile artist, capable of understanding a wide range of model types, including black-box models. It’s like a skilled improviser, exploring different input variations to reveal how they influence predictions.
SHAP (SHapley Additive exPlanations)
It excels in precision, meticulously attributing credit to each feature for its contribution to the prediction. It’s like a conductor carefully balancing the contributions of each instrument, working best with models based on linear relationships or interpretable features.
Conducting the XAI Symphony
The art of XAI lies in selecting the right techniques for the task at hand and harmonising them to create a comprehensive understanding of AI’s decision-making. By combining model-specific and model-agnostic approaches, model-centric and data-centric perspectives, and leveraging the strengths of techniques like Lime Explainable AI and SHAP, we can transform AI from a mysterious oracle into a transparent and trusted partner.
Real-World XAI: A Case Study in Demystifying Mortgages
Remember those detective novels where a seemingly airtight case unravels thanks to a single clue? Explainable AI (XAI) is our modern-day Sherlock Holmes, shedding light on the opaque world of AI decision-making. Let’s delve into a real-world example: using XAI to demystify mortgage approvals.
Unlocking the Black Box
Imagine applying for a home loan, your future hinging on an algorithm’s enigmatic “yes” or “no.” You submit your details, your credit score gleaming like a trophy, yet the rejection letter arrives, shrouded in algorithmic secrecy. Frustration and confusion simmer – was it the income gap, the student loan ghost, or the mysterious credit card you barely use?
Enter LIME (Local Interpretable Model-agnostic Explanations), our friendly financial advisor for AI models. It acts like a Sherlock on the case, asking the model, “Hey, what factors pushed this applicant’s file towards rejection?” Lime Explainable AI then dissects the model’s inner workings, highlighting the specific elements that tipped the scales against the borrower.
Revealing the Clues
The results? Intriguing, to say the least. Perhaps it wasn’t just the income, but the combination of income and a recent job change that raised the model’s eyebrow. Or maybe, it was the high utilisation of that rarely used credit card, suggesting potential financial instability. Whatever the reason, LIME unmasks the mortgage mystery, providing the borrower with valuable insights.
And this is how Explainable AI (XAI) works – helping you unmask the reasons behind why AI took certain decisions in certain scenarios.
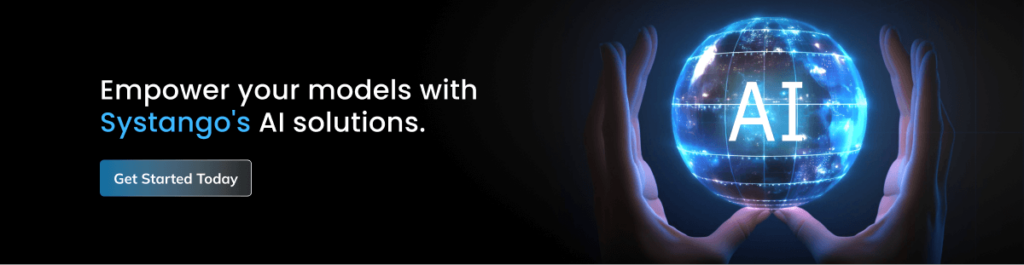
Beyond Individual Cases: 5 Powerful Explainable AI Use Cases
Explainable AI (XAI) is no longer a sci-fi dream, but a vital tool illuminating the often-murky world of AI decision-making. Let’s explore five powerful Explainable AI use cases where XAI can revolutionise diverse fields:
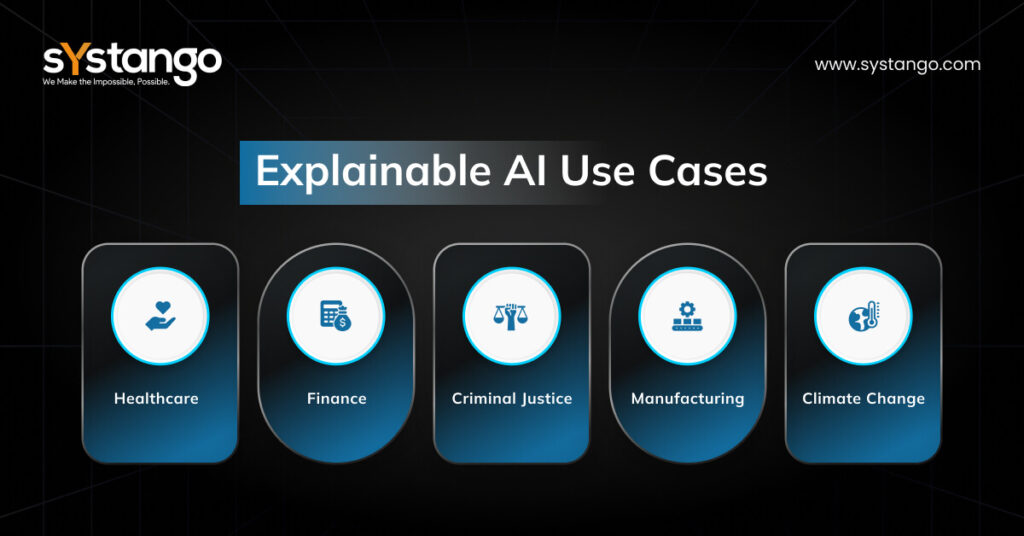
1. Healthcare
Imagine diagnoses explained not as cryptic codes, but as clear insights on influencing factors. XAI empowers doctors with explainable medical diagnoses, optimising resource allocation, streamlining drug development, and fostering trust with patients.
2. Finance
Unravel the mystery of loan approvals! XAI sheds light on credit risk assessments, ensuring fairness and transparency for borrowers. Imagine XAI explaining loan decisions, allowing financial institutions to tailor recommendations and build trust with customers.
3. Criminal Justice
Predicting crime rates without perpetuating biases? Explainable AI analyses data to inform risk assessments, optimise resource allocation, and even detect potential bias in algorithms. By explaining crime predictions, XAI can promote fairer sentencing and resource allocation.
4. Manufacturing
Predict equipment failures before they happen! XAI analyses sensor data to explain potential machine downtime, enabling proactive maintenance and optimising production lines. With clear explanations for predictions, manufacturers can make informed decisions to protect their operations.
5. Climate Change
Understand the complex web of factors driving climate patterns. XAI helps analyse climate data, offering clear explanations for predictions and enabling scientists to develop impactful mitigation strategies. By demystifying complex models, XAI empowers informed decision-making for a sustainable future.
Another use case can be AI and Data Analytics wherein XAI can be extremely beneficial. These are just a glimpse into the potential of XAI. As it evolves, Explainable AI Use Cases will continue to expand, illuminating the path towards a fairer, more transparent future powered by intelligent technology.
The Future of Explainability
The future of Explainable AI, dear reader, promises to be as exciting as a sci-fi thriller. Here are some glimpses into this crystal ball:
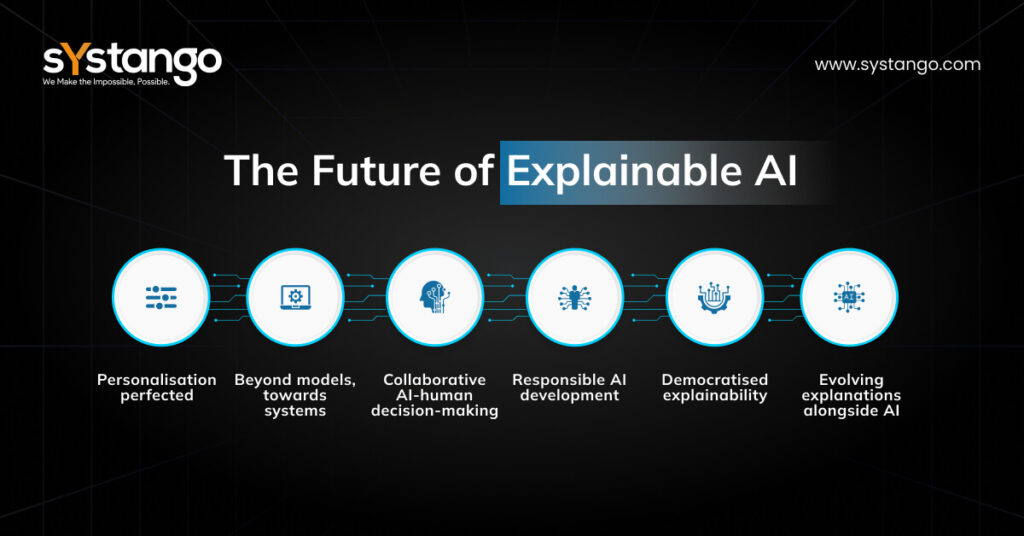
1. Personalisation perfected
Explanations tailored to individual users, not dry technical jargon. Imagine mortgage feedback presented as clear action steps like “Reducing credit card utilisation can boost your approval chances”.
2. Beyond models, towards systems
XAI will delve deeper, examining entire AI systems, not just individual models. This holistic approach will ensure transparency and fairness across the entire chain of command.
3. Collaborative AI-human decision-making
Explainable AI will bridge the gap between human and machine intelligence, fostering trust and allowing humans to guide AI decisions with informed insights.
4. Responsible AI development
Ethical considerations will be woven into the fabric of XAI, ensuring explanations are not misused and AI technology aligns with human values.
5. Democratised explainability
User-friendly XAI tools will empower everyone to understand AI, not just tech experts. Imagine checking your insurance coverage with an XAI app, easily grasping the factors influencing your premiums.
6. Evolving explanations alongside AI
As AI models progress, so will XAI methods. This dynamic dance will ensure explanations remain relevant and accurate, keeping pace with the ever-evolving world of AI.
The future of XAI is not just about opening the black box of AI, but about building a bridge between humans and machines. It’s a future where trust, fairness, and understanding pave the way for a more equitable and collaborative world powered by intelligent technology.
End Words: Your Turn to Investigate
The curtain has been raised on the once-opaque world of AI, and very importantly XAI. We have analysed in depth what XAI is and why it is important today. With Explainable AI, we’ve glimpsed the gears and levers driving those enigmatic algorithms, a feat akin to cracking the Da Vinci Code for machine learning. But unravelling the mystery is only the first act. Now, it’s about forging a future where AI thrives in the sunlight of transparency, accountability, and trust.
This is where Systango steps in, your trusty Watson in the age of intelligent machines. We offer a potent toolkit of XAI solutions, not just to illuminate AI decisions, but to guide them towards responsible, ethical outcomes. Imagine loan approvals explained not with cold percentages, but with clear pathways to improvement. Picture healthcare diagnoses not as cryptic pronouncements, but as actionable insights empowering patients and shaping better outcomes. This is the future Systango helps you build, a future where AI doesn’t just make decisions but explains them, collaborates with us, and ultimately earns our trust.
Ready to demystify your own AI and write a new chapter in human-machine collaboration?
Let’s illuminate the path together. The future of AI is transparent, it’s responsible, and it’s yours to shape.
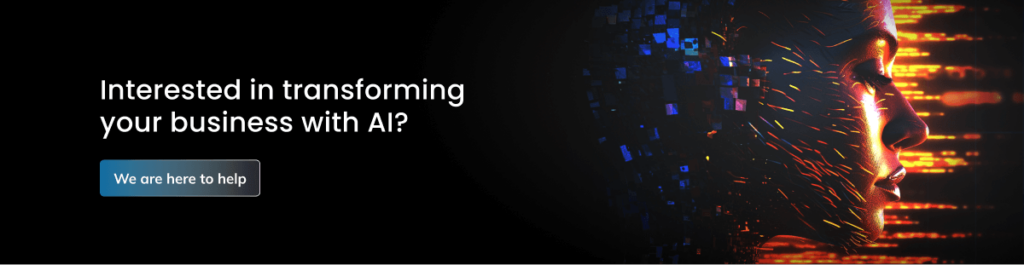